Research Data Management
Research Data Management (RDM) describes the organisation, storage, preservation and sharing of research data collected and used in a research project. The key elements of RDM include:
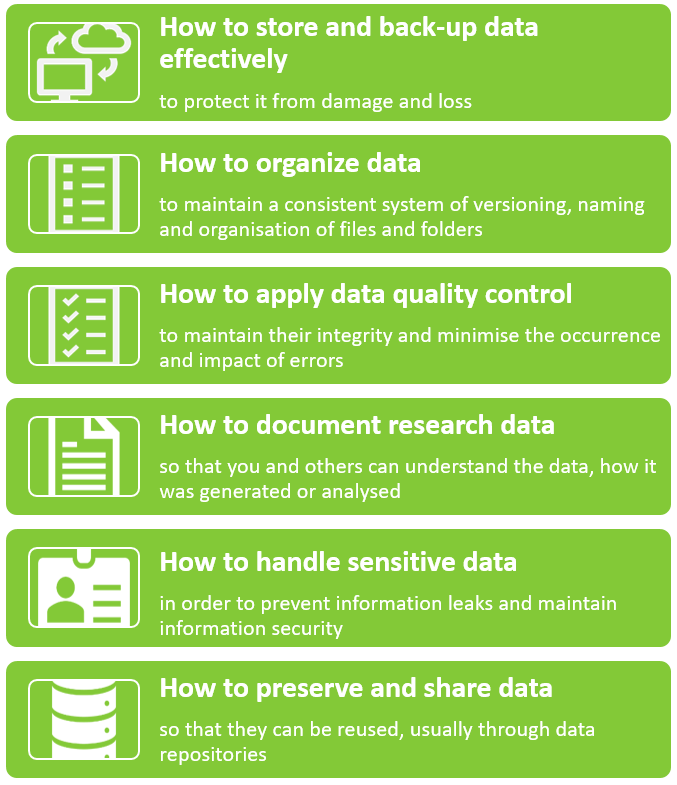
Advantages of research data management
Data is an essential research capital that allows you to get answers to research questions but also serves as a source of information to validate the results of your scientific work. Research data is a very valuable resource that can be reused in your own research, and in the research of the wider scientific community. Moreover, if your research data is reused in this way, it can be cited, increasing your academic performance.
Active research data management:
- Makes work easier and saves time: well-organized data management will increase your efficiency and save you time and effort in the long run when working with data;
- Protects you and other researchers: reducing the risk of unpleasant incidents such as data loss or confidential data leaks;
- Preserves the integrity of your research: well-documented data demonstrates the authenticity of the research and the reliability of the findings;
- Highlights the value of research data: data that is preserved and accessible over the long term can be reused for your benefit and the benefit of others.
RDM starts with planning data management. Research funding agencies often include research data management practices in their conditions for providing research funding. Considering these reasons, creating a data management plan (DMP) for every conducted research or project based on a collection of primary data is good practice.
Data life cycle
While creating a data management plan for your research project, it is advisable to look at the research data through the data life cycle. It can help to ensure different steps of planning research will be included during data analysis or when sharing the data.
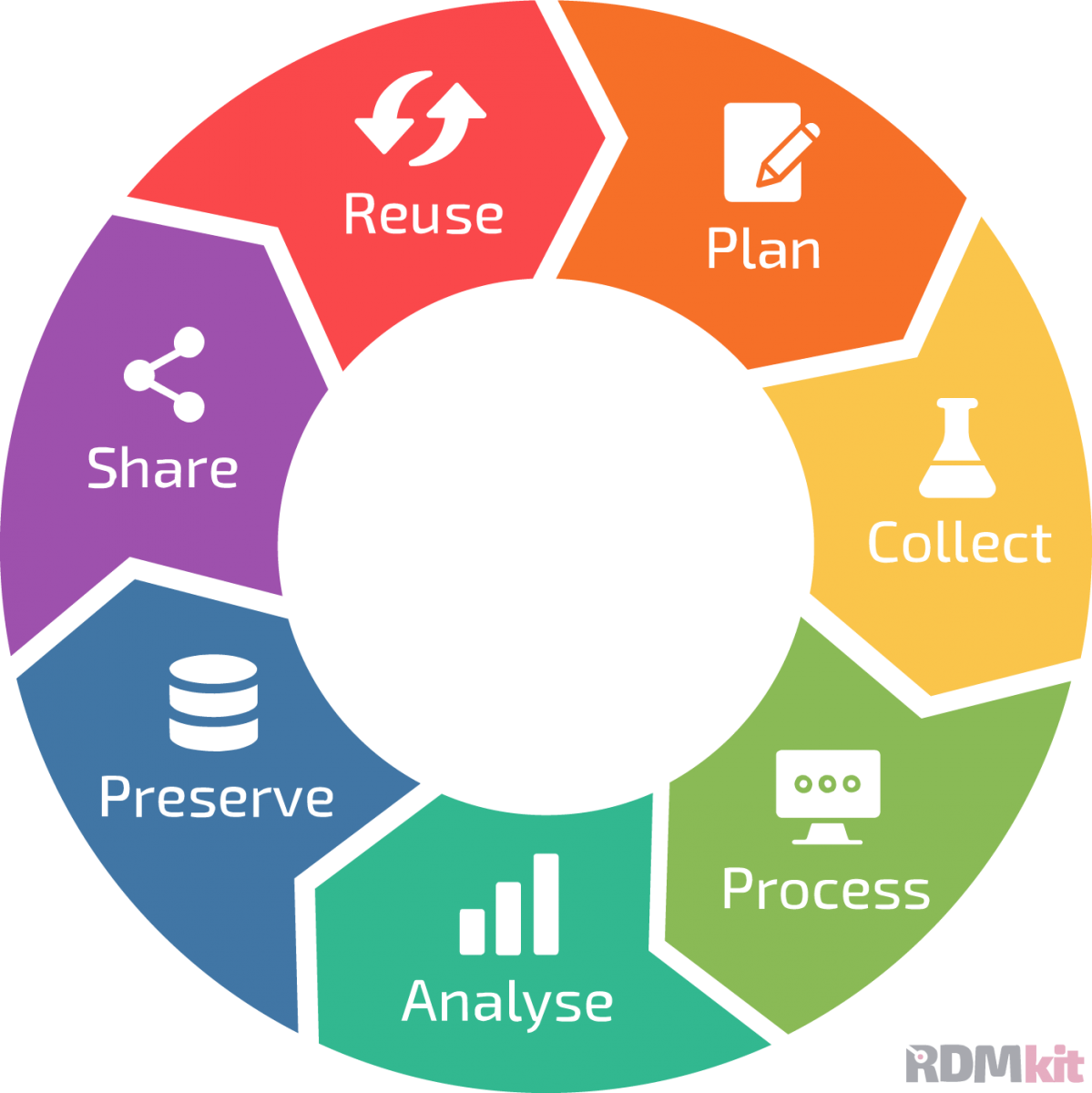
RDMkit by ELIXIR-CONVERGE, Creative Commons Attribution 4.0
Research data management based on Data life cycle
1. Planning
In this part, you make sure what data will be collected and used in order to answer your research questions and plan the research data management for the complete data life cycle. During this phase, the data management plan is created. The number of research funding agencies requiring data management plans to be a part of interim reports is increasing. Further, it is necessary to set:
- File naming convention
- Folder naming convention
- System of organizing folders
- Data documentation and creation of ReadMe file/files
- Storage for data processed during the research
- Choose a data repository for long term storing of research data after the end of the project
- License for data published in the data repository after the project
2. Data Collection
In this phase experiments, observation and surveys are conducted, and more secondary data is obtained together with other materials. This phase should include active documentation of tools and methods of data collection, recording information crucial for the data interpretation and data re-use. Regular updates to the data management plan are necessary.
3. Data Processing
To answer the research questions, it is necessary to analyse and interpret the collected data. This process might include data cleaning, combining datasets from different sources (e.g., format conversions) and use of processes of validation and data quality control. Any processing of your research data must be documented so that the final result can be replicated and verified.
4. Data analysis
Methods used for the analysis should be documented and specifically described in the data management plan. Do not forget to describe specific formats of files, and data volume, or to include the name of the software, you have used to analyse the data.
5. Data Preservation
Once you have completed your research, you should keep for long-term use research data which documents the results of your research and has long-term value. The data will need to be prepared for preservation and archived in a suitable location. In many cases, this will involve storing the digital data in a suitable data repository. Preservation activities may include ensuring data quality, converting file formats to open forms, , creating metadata records with Digital Object Identifiers (DOIs) assigned to datasets, licensing datasets for reuse, and implementing any required access controls.
Highly valuable non-digital data may be stored locally, in which case it should be managed by a responsible person or group who can ensure its proper storage and preservation.
6. Data Sharing
Publications based on research data should include a citation, link, or persistent identifier DOI, or data availability statement explaining under what conditions the underlying data can be obtained. Data repository ensures open availability of metadata online and enables access to data under conditions of assigned license. Data can be open to the public or can be published with restricted access, under a time embargo (for example till your scientific article is published).
7. Data Re-use
Open data published in a data repository can be reused by other researchers and institutions, or serve as verification of your research results. Re-use of data can lead to the discovery of new research findings and can serve as a valuable source for the creation of policies, and development of new commercial products, or can serve as an educational tool.
How FAIR is your data?
FAIR principles refer to good practice of research data management. It aims at increasing findability, accessibility, interoperability and re-usability of research data:
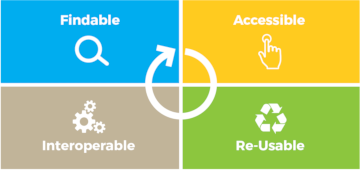
St. Lawrence Global Observatory. 2024. FAIR Principles. [cit. 24-02-28]. https://ogsl.ca/en/fair-principles
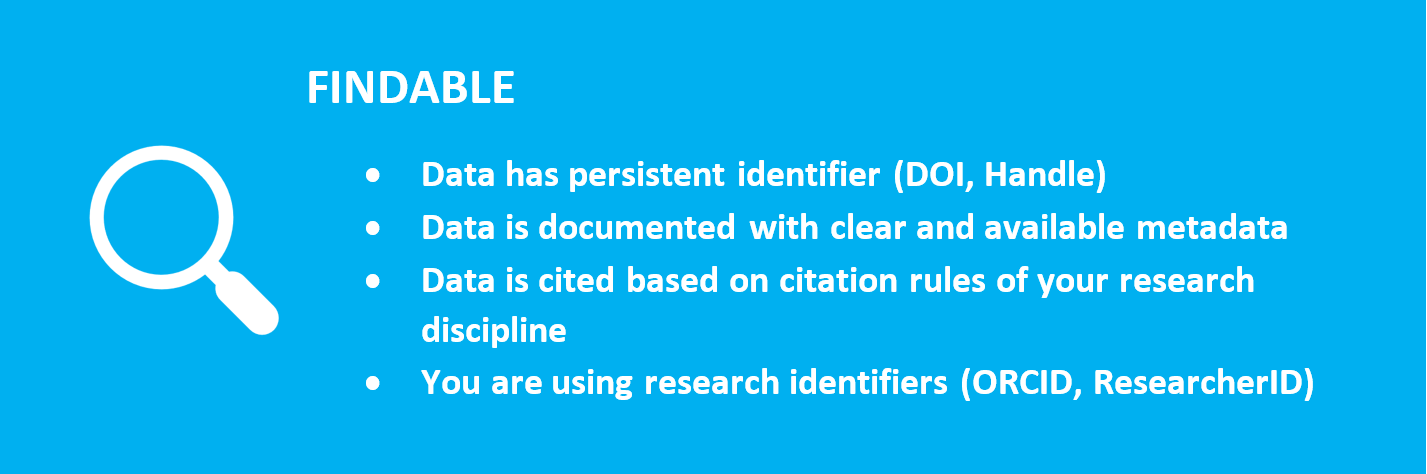
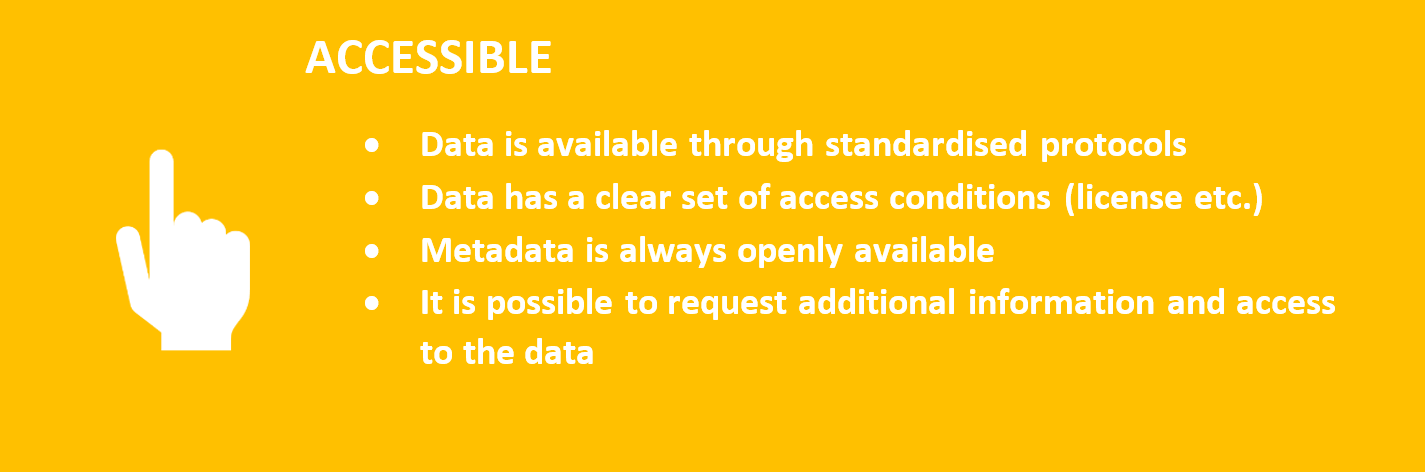
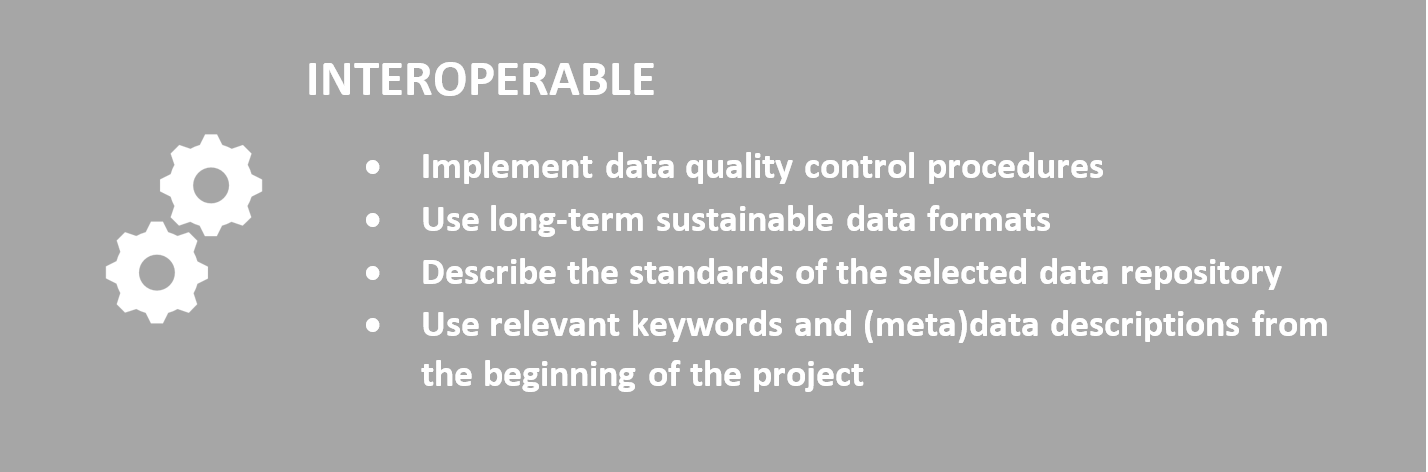
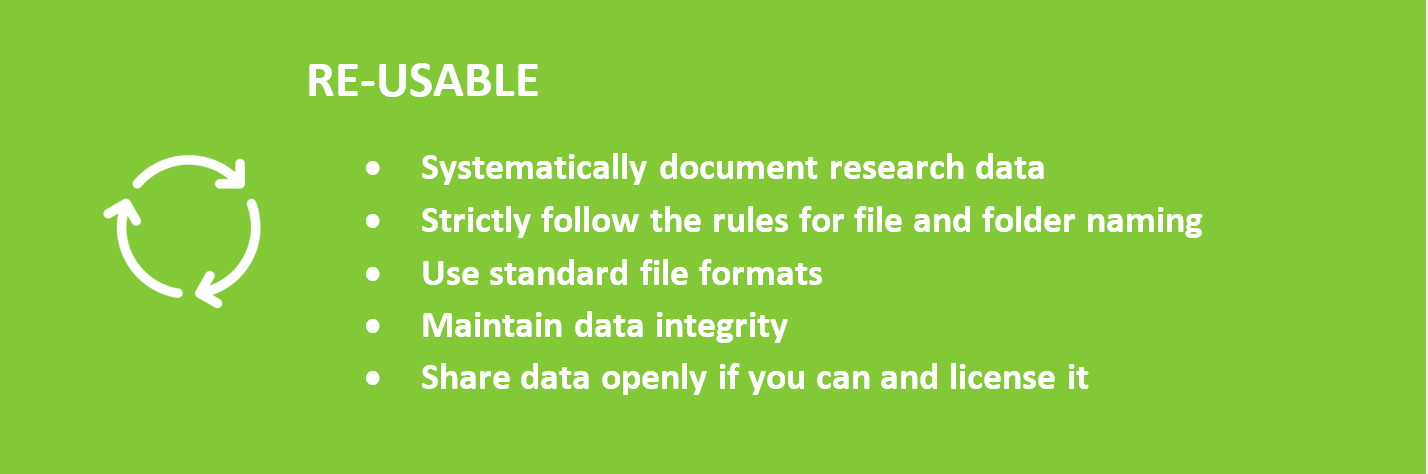
- FAIR data does not automatically mean open data. The rule: As Open as Possible, as Closed as Necessary always applies.
- Check how FAIR is your data. Some projects require information on how your data will comply with FAIR principles.
Additional Materials and Links
References
- RDMkit. 2024. Data life cycle. ELIXIR-CONVERGE [cit. 24-02-27]. https://rdmkit.elixir-europe.org/data_life_cycle
- St. Lawrence Global Observatory. 2024. FAIR Principles. [cit. 24-02-28]. https://ogsl.ca/en/fair-principles
- University of Reading. 2024. The research data life cycle. [cit. 24-02-28]. https://www.reading.ac.uk/research-services/research-data-management/about-research-data-management/the-research-data-lifecycle
- University of Reading. 2024. What is research data managment. [cit. 24-02-28]. https://www.reading.ac.uk/research-services/research-data-management/about-research-data-management/what-is-research-data-management