Data categorization
Data categorization enables the differentiation of various types of research data based on the level of their sensitivity or value for the research organization/research, in case the data would be changed, destroyed or even stolen. Data categorization helps researchers understand the value of analysed research data and decide what control and security measures are necessary to implement to prevent potential risks.
Research data can be separated into four different categories based on their sensitivity. Categorizing data as a specific category later impacts how the data is stored, what security measures are applied, or even the option for uploading the data into the data repositoryonce the research is finished.
PUBLIC DATA | |
Data is accessible without any restrictions to anyone.
An example is data publicly available on the internet. Its publication does not endanger/harm anyone. |
|
Suitable storage locations: Physical Storage ; Cloud solutions (external) ; Network Drives (internal) ; Cloud Solutions (Contractual) | |
Data can be published in a data repository without restrictions |
INTERNAL DATA | |
Does not require special regulation or protection by law/contract.
Data is meant for internal use of an organization/group of people. Disclosure outside the group will not cause direct harm (financial, moral, legal). |
|
Suitable storage locations: Physical Storage ; Network Drives (internal) ; Cloud Solutions (Contractual) | |
Publishing data in a data repository is possible after consideration |
DISCRETE DATA | |
Data requiring regulation or protection, typically data that is protected by law or under a contract/licence.
Data intended exclusively for the internal use of a well-defined group of persons, e.g., an employee and his/her supervisor, an employee of the HR department and a job applicant, a group of IT system administrators with administrative rights to the system. This is categorized as personal data, data covered by trade secrets. Disclosure of the data outside an identified group of persons is very likely to cause damage (financial, moral, legal...). |
|
Suitable storage locations: Network Drives (internal) ; Cloud Solutions (Contractual) | |
Uploading to a data repository cannot be done without restrictions* |
SENSITIVE DATA | |
By their nature, they require special regulation or special protection, typically the data is strictly protected by law or by contract/license.
Data intended strictly for internal use of a well-defined group of persons, for example, a health care professional and a patient, project developers with a certain level of security clearance. This is very valuable data falling under trade secrets, sensitive personal data. Disclosure outside a given group of authorised persons will cause damage (financial, moral, legal) on a large scale with serious consequences. |
|
Suitable storage locations: Network Drives (internal) ; Cloud Solutions (Contractual) | |
Publishing data in a data repository is not possible without restrictions and other measures* |
The content of the page was inspired by the page of Masaryk University: Recommendations for the Usage of Storages
Some categories of research data cannot be made publicly available without restriction once the research is completed, precisely because of their nature. This applies in particular to the category of sensitive data. Researchers should takeadditional precautions, in accordance with relevant security, ethical and legal regulations, before planning to release sensitive data.
Recommended measures for the handling of sensitive data during and after the research:
1. Informed consent
PBefore starting your research project or even submitting the grant application, it is necessary to clearly set the way data will be collected/generated. In ideal case, you should inform institutional Human Research Ethics Committee about your research design and plan for sharing the sensitive data after finishing your research project. Timely creation of the plan for storing and sharing sensitive research data is necessary for informing potential research participants about your intents to share the sensitive data and also to obtain their informed consent
Already when recruiting participants, you should inform all participants about the research topic, your plan for storing their sensitive data during the research, and your plan for sharing them after your work is finished. Informed consent must be obtained in written or at least oral form (recorded in .mp4) and must be obtained based on participants free will – e.g., it is not possible to create a form with already pre-ticked boxes. Participants must also be able to withdraw from the research if they disagree with the way their data is stored and shared.
2. Anonymisation
Anonymisation removes identifying information from the dataset. It serves as a tool for protecting privacy of research participants and reduces probability of re-identification. Despite the removal of identifying information from the dataset, anonymisation can be applied only after informing participants and obtaining their consent.
When considering anonymization of the dataset you will have to probably remove direct and indirect identifiers. Direct identifiers, for example, full name, date of birth, or address, can clearly identify the research participant. Indirect identifiers can indirectly in combination with other information from the dataset, clearly identify the research participant, e.g., ethnic origin, race, sex, place of birth, or work position. The process of anonymization will be always dataset-specific which is why a universal step by step guide cannot be created. During the anonymisation process, you should regularly check the data files with sensitive data, to make sure you have already removed enough sensitive data without damaging the value of the dataset.
The main techniques of anonymisation include:
- Removing variables which are not necessary for the analysis or not relevant to the research
- Generalisation: reducing the specificity of information, for example substituting a city for an address.
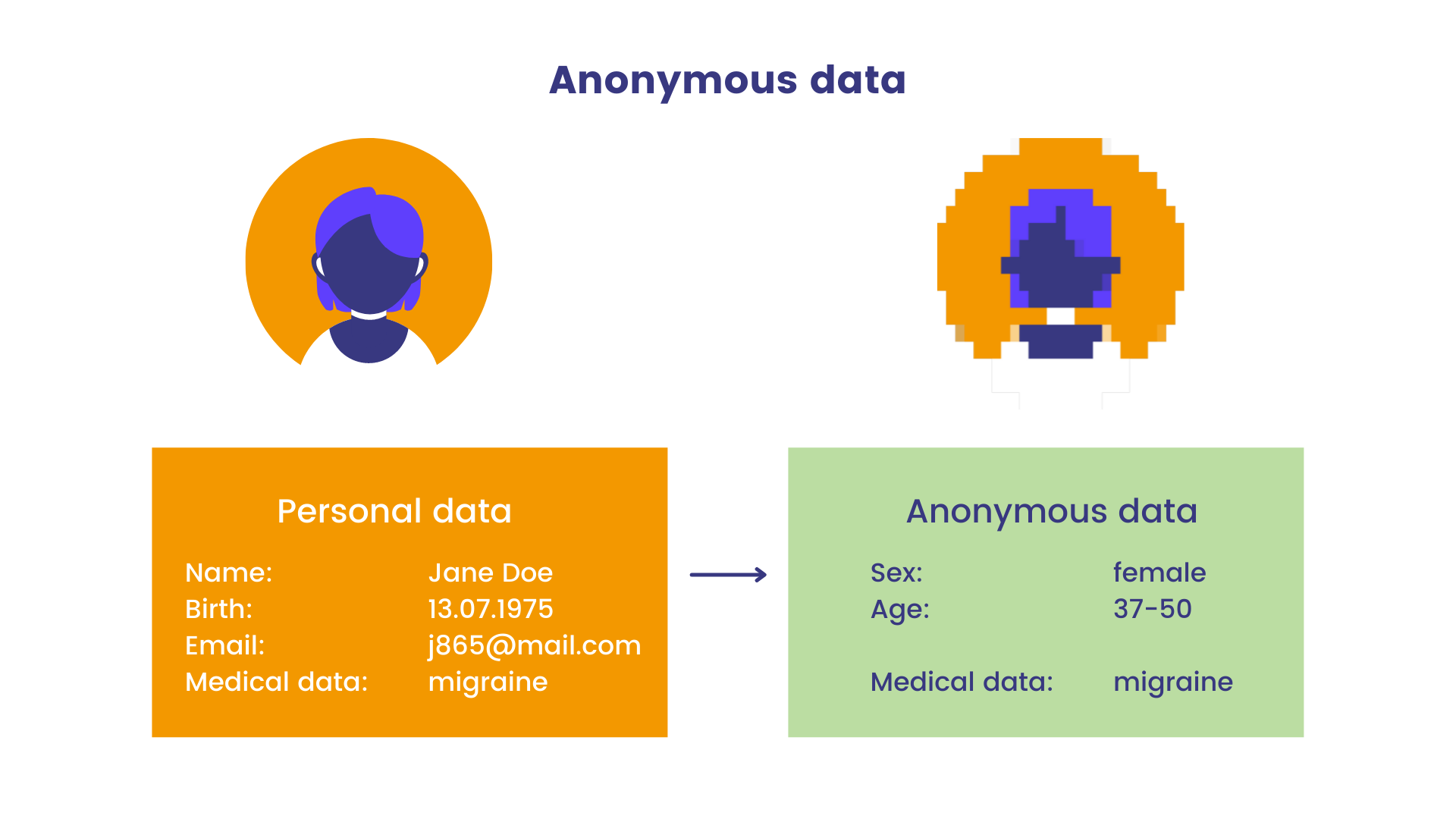
Statice. 2023. Infographics. In: Pseudonymization vs anonymization: differences under the GDPR. [cit. 24-02-27]. https://www.statice.ai/post/pseudonymization-vs-anonymization.
- Pseudonymisation: referring to a research participant without using their actual data by substituting their name for another created version.
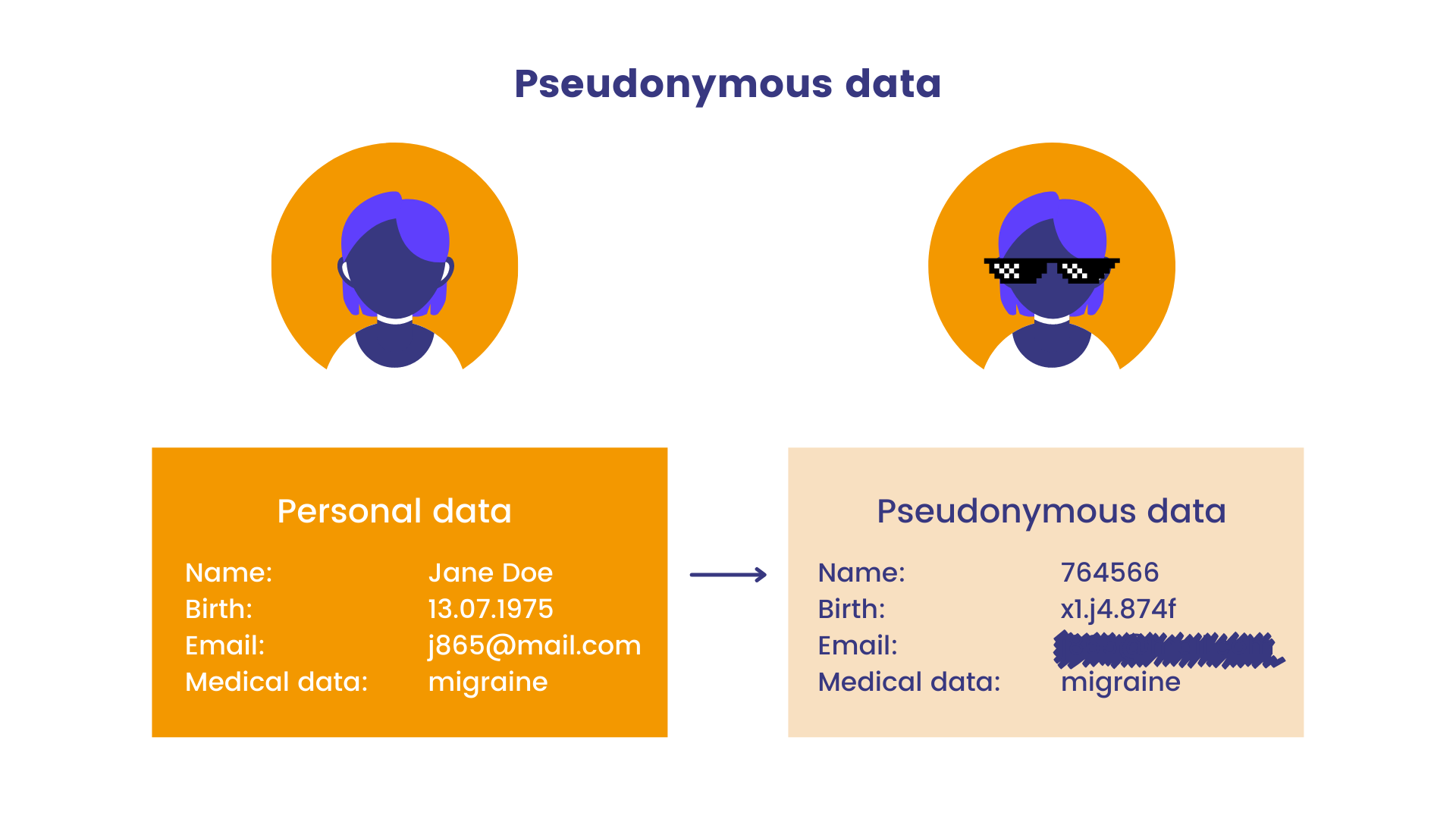
Statice. 2023. Infographics. In: Pseudonymization vs anonymization: differences under the GDPR. [cit. 24-02-27]. https://www.statice.ai/post/pseudonymization-vs-anonymization.
- Banding: taking specific information, such as age, and putting it into a range
When anonymising one dataset, one may have to apply all of the described techniques. Similar techniques can be used for anonymisation of qualitative data and any change to the original data can be labelled with diacritics around the text, e.g., # or @.
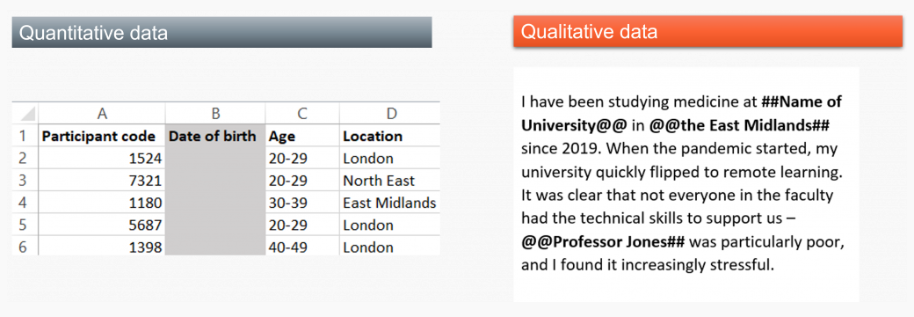
F1000 BlogNetwork. 2019. Sharing sensitive data: key consideration and approaches for safer sharing. [cit. 24-02-27]. https://blog.f1000.com/2023/01/12/safe-sharing-sensitive-human-research-data
3. Controlled access
In cases, when your datasets or files with sensitive data cannot be fully anonymised, or when the process of anonymization has led to the devaluation of the data and impaired its usability in research, it is possible to publish the data in a data repository with controlled access, however, only under condition of consent of all research participants.
Data repositories with controlled access offer a place where researchers can share datasets, but do not open them publicly. Usually, instead of sharing all data openly, only metadata describing the record is open and the repository ensures the dataset can be access only by approved users. If you publish scientific work based on a sensitive data and your dataset was uploaded to a data repository with controlled access, you can use section Data availability statement in your manuscript to link to the data and explain under what conditions will be available. More information about data repositories with controlled access can be found on tab Data repositories.
References
- F1000 BlogNetwork: https://blog.f1000.com/2023/01/12/safe-sharing-sensitive-human-research-data
- Evropská komise: https://commission.europa.eu/law/law-topic/data-protection/reform/rules-business-and-organisations/legal-grounds-processing-data/sensitive-data/what-personal-data-considered-sensitive_cs
- Statice: https://www.statice.ai/post/pseudonymization-vs-anonymization